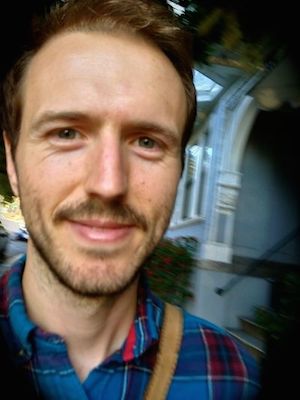
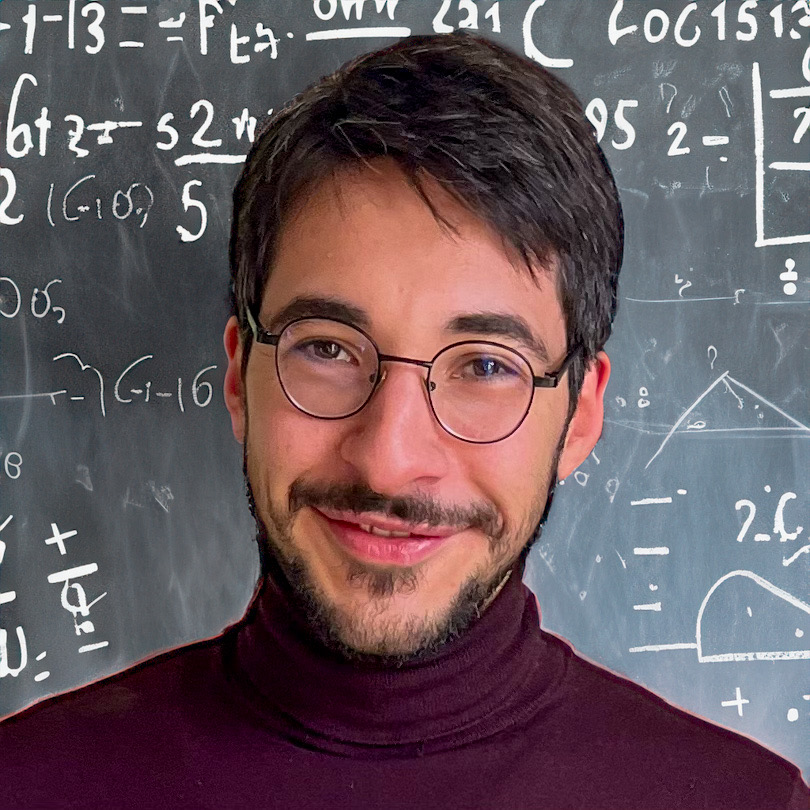
A core challenge in machine learning is understanding how neural networks construct internal representations of data structures. But what exactly constitutes data structure in this context? How can we develop simple yet insightful mathematical models to describe it? How does the learning algorithm encode these structures, and how does the amount of training data influence this process? These questions are central to my research, which combines theoretical approaches — inspired by methods from statistical physics — with controlled numerical experiments. Currently, my work focuses on generative AI models, particularly diffusion models, and formal grammars from linguistics. By exploring these models, I aim to uncover the principles that govern how deep learning algorithms represent and generate structured data.
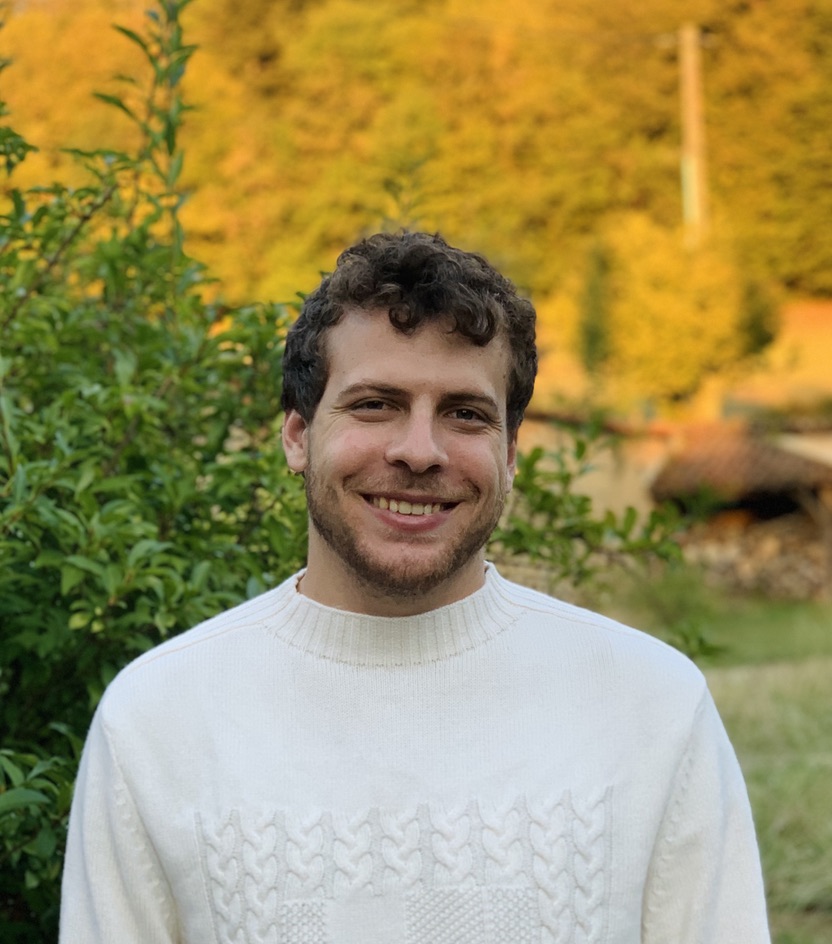
Brains have a seemingly infinite ability to learn, remember and forget. As a proxi for learning in the brain, I study the learning rules that unlock such flexible and robust learning in neural networks models. I am particularly interested in the relative contributions and interplay between the various processes thought to be involved in biological learning, for instance synaptic plasticity, structural plasticity and innate connectivity motifs. To understand how these processes may be implemented in the brain, I use tools from Physics and ML, combining top-down (e.g. analytical derivations in linear recurrent networks) and bottom-up approaches (e.g. numerical simulations/optimizations of rate or spiking recurrent networks).
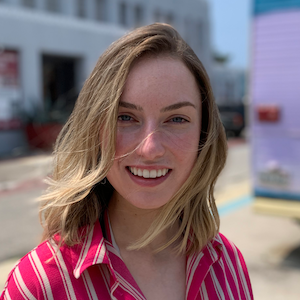
I am interested in how intelligent agents can make rich and structured inferences from impoverished data. I demonstrate how such abilities can be implemented via learning algorithms in neural networks, with the aim of grounding higher-level cognitive phenomena in a candidate neural implementation. This research requires me to use cross-disciplinary insights from psychology, neuroscience, and machine learning, in addition to a combination of behavioral experiments, computational simulations, and analytical techniques.
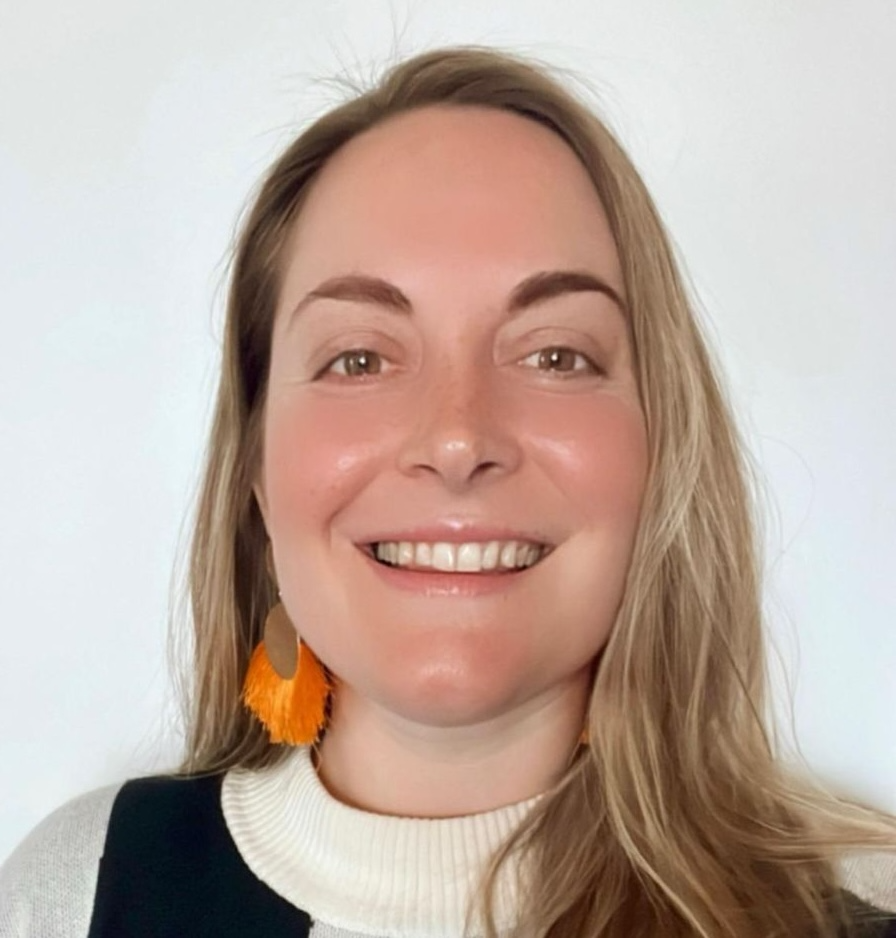
A remarkable feature of the brain is its ability to transform experience into structured knowledge that enables open-ended learning - something current machine learning systems still struggle to achieve. Sleep plays a critical role in this process by adaptively reshaping neural representations, yet how the neural dynamics unique to sleep accomplish this remains poorly understood. My research aims to advance our understanding of sleep function through cross-disciplinary insights from neuroscience and machine learning, with a current focus on modeling systems consolidation between the hippocampus and neocortex at both mechanistic and normative levels of abstraction. This work is motivated by a broader interest in “local–global scaling problems”.
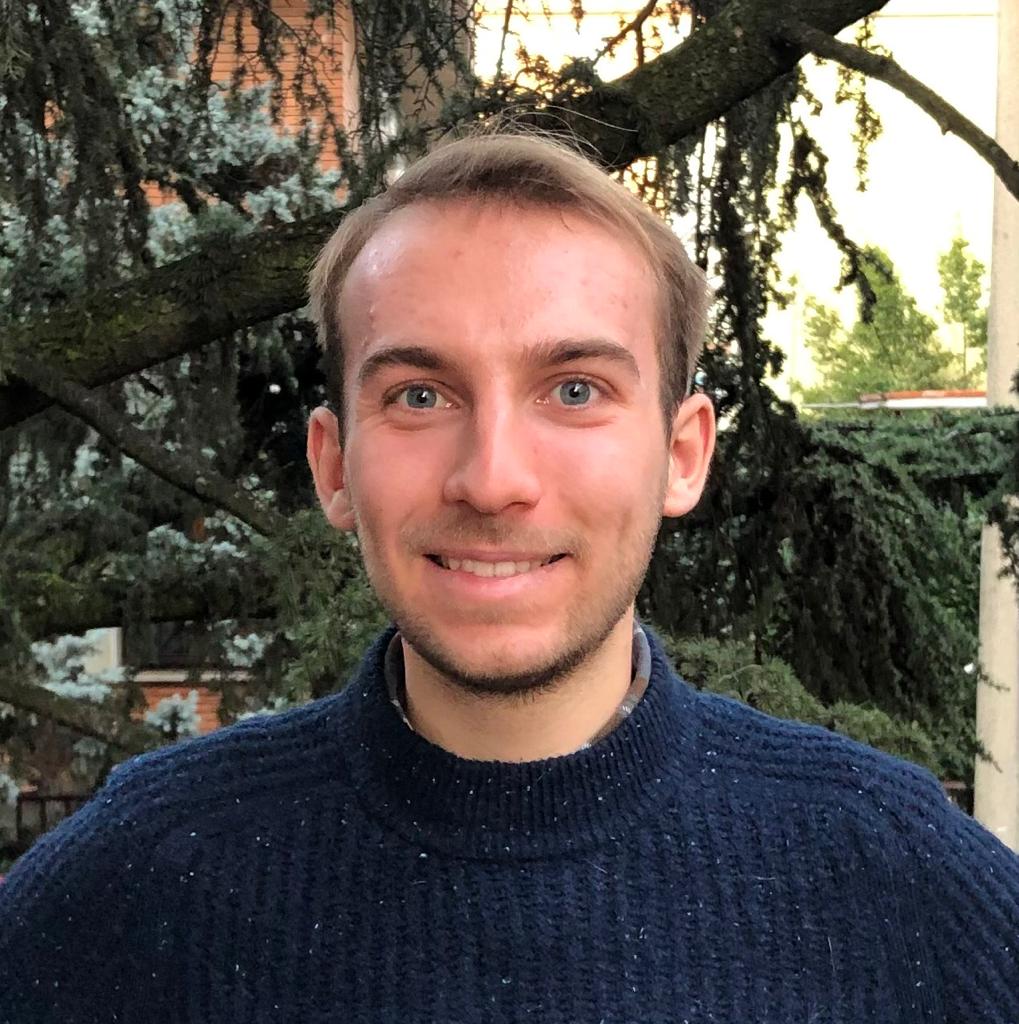
Learning over long timescales often involves developing increasingly effective strategies or learning multiple skills. In addition, we frequently learn in social settings where individuals can specialise differently and collectively solve complex problems. My research investigates such individual and collective long-term learning from both theoretical and experimental perspectives.
I am a Research Fellow in Theoretical Neuroscience at the Gatsby Computational Neuroscience Unit (UCL) funded by the Human Frontier Science Program (HFSP) and Gatsby Charitable Foundation.
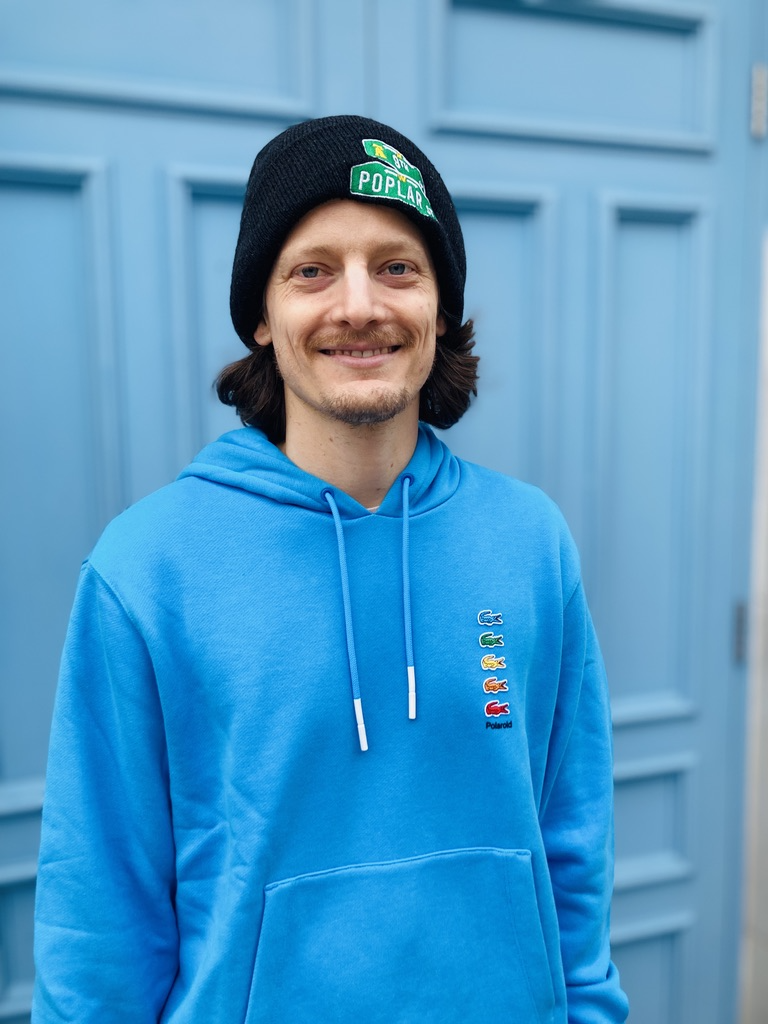
How does the brain rewire itself in response to experience? We think that learning in the brain proceeds by changing the connection strengths between neurons, but what are the rules that govern this process? What kinds of representations do they produce? And how do these representations support adaptive (and maladaptive) generalization of previously learned relationships? My research aims to address these questions by studying the behavior of rodents and their patterns of neural activity as they learn to perform complex tasks with richly structured associations.
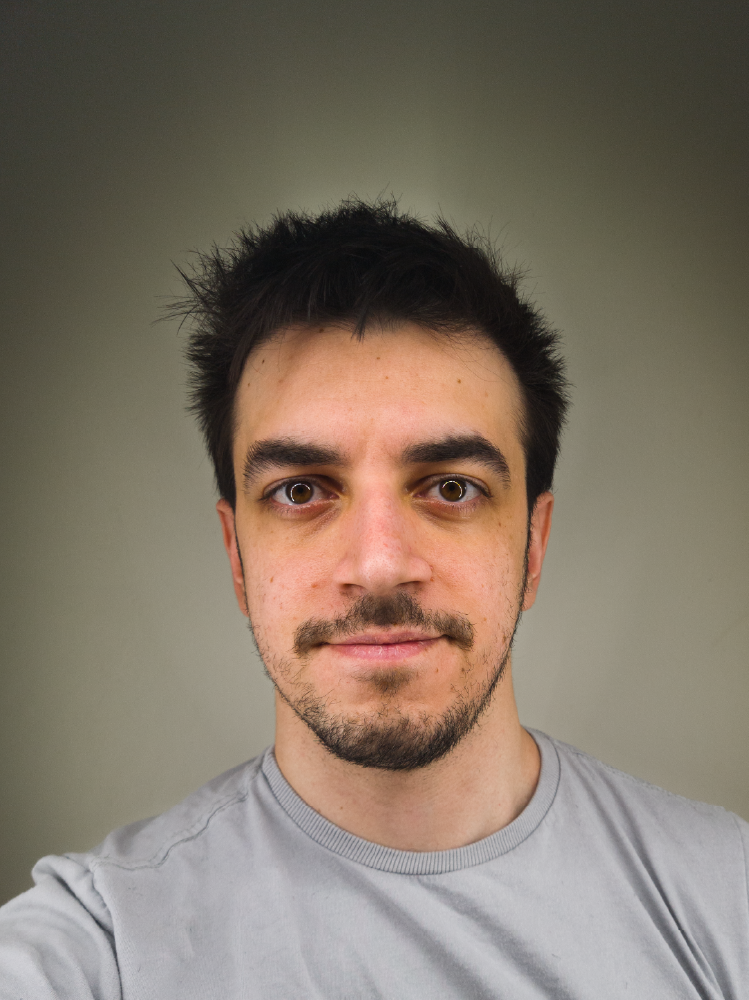
I earned my PhD from Imperial College London under the supervision of Claudia Clopath. During my doctoral studies and subsequent postdoctoral position, I focused on developing mechanistic models of synaptic plasticity. Seeking to further collaborate with experimental researchers, I joined Athena Akrami’s lab at the SWC where we developed a cross-species research project investigating decision-making in rodents, humans, and computational models. Recently, I joined the Saxe lab to delve deeper into the intersection between experimental and theoretical neuroscience, with a particular interest in the mechanisms and fundamentals underlying learning and memory formation.
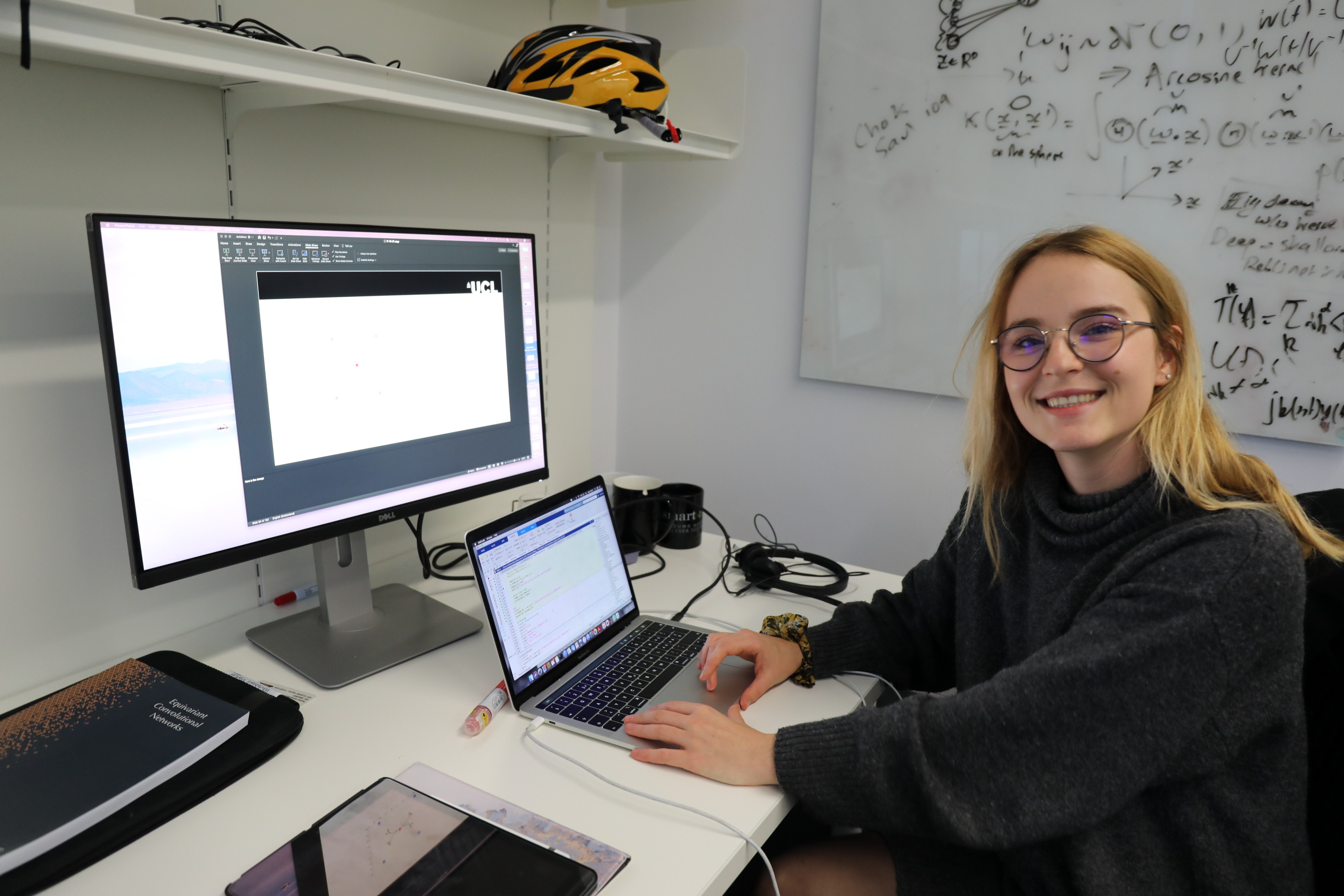
I am particularly interested in studying the computational neural theories at the basis of learning and memory consolidation in neuronal networks. I am interested in working toward answering questions such as: How does the brain create, store, generalize and update memories without interfering with previously stored memory? What is the function of episodic memory? I am looking forward to making advances in answering these questions, working at the intersection between theoretical neuroscience research and machine learning.
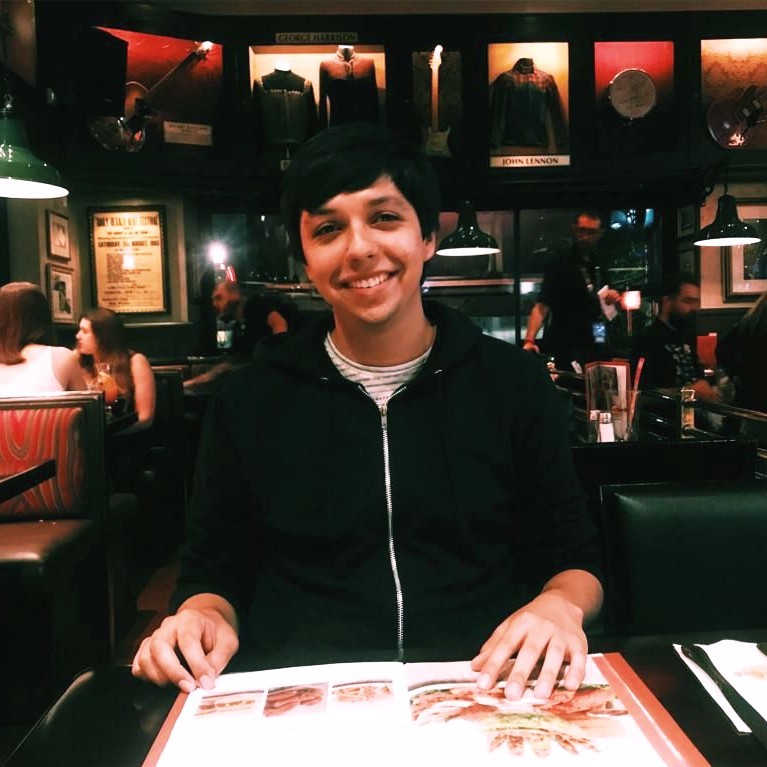
Whether a novel task is worth learning, how effort may impact learning, and how much effort to allocate towards learning are important questions agents face. To answer these questions, I’m currently working in models of cognitive control for learning systems. Particularly how control signals might shape the learning dynamics in linear networks. I’m also collaborating with Clementine Domine (somewhere in this same page) to build a software for people to test their models on hippocampus and entorhinal cortex, on simulated environments that somehow resemble the experimental setting, to directly compare with neural data recorded from each experiment. I have a broad set of interests, such as neuroscience, machine learning, biology, and philosophy in science. Feel free to reach if you want to chat!
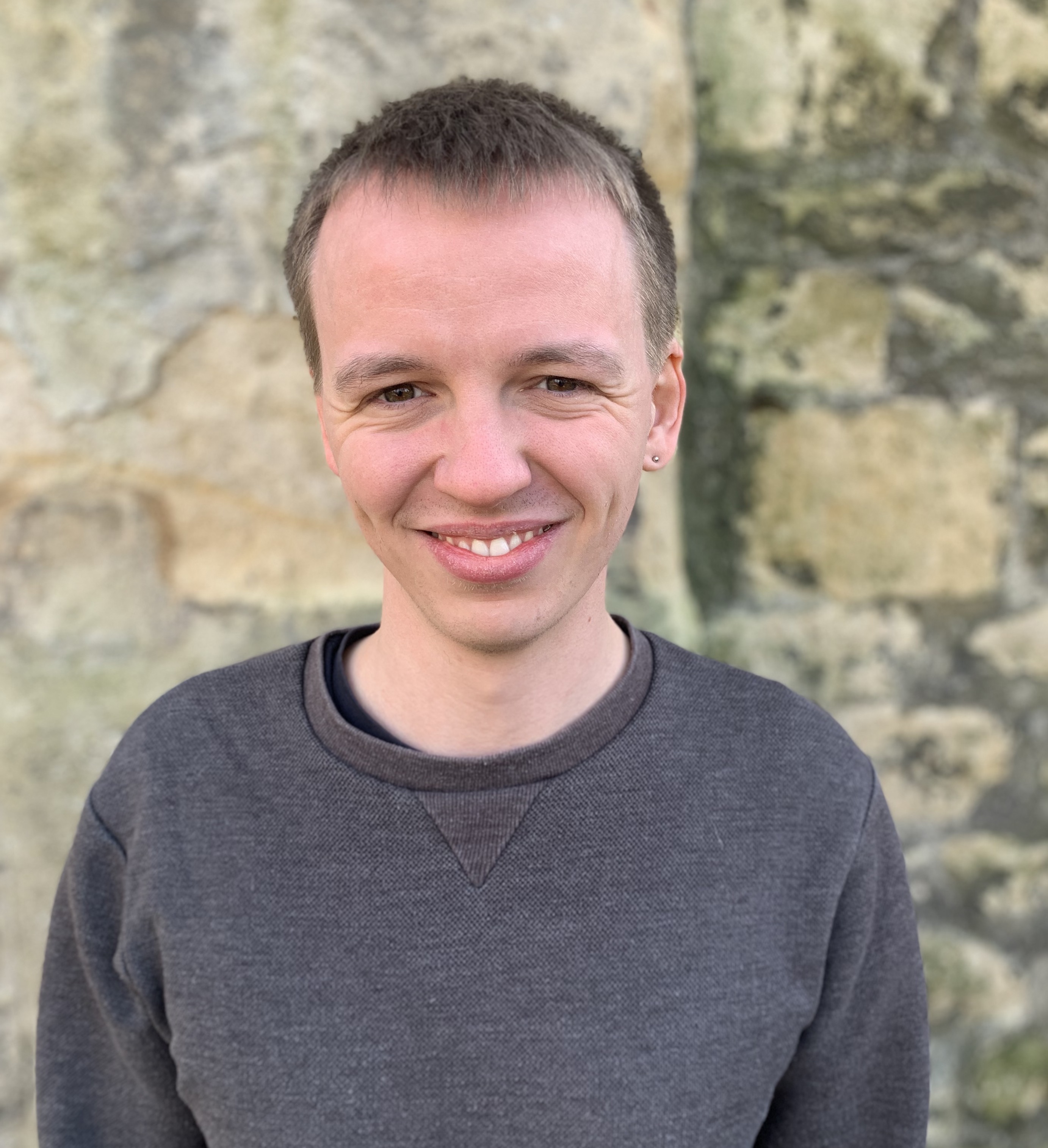
I am a student on the GUDTP 1+3 in Experimental Psychology at the university of Oxford co-advised by Chris summerfield and Andrew Saxe.
My interests range from Cognitive Neuroscience and Psychology to Computational Neuroscience and Machine Learning. My MSc work investigates semantic learning. Specifically, I will examine if behavioural and representational changes during the learning of semantic knowledge are analogous to those observed in deep linear networks. To achieve this end, we employ behavioural experiments, neuroimaging, and modelling experiments.
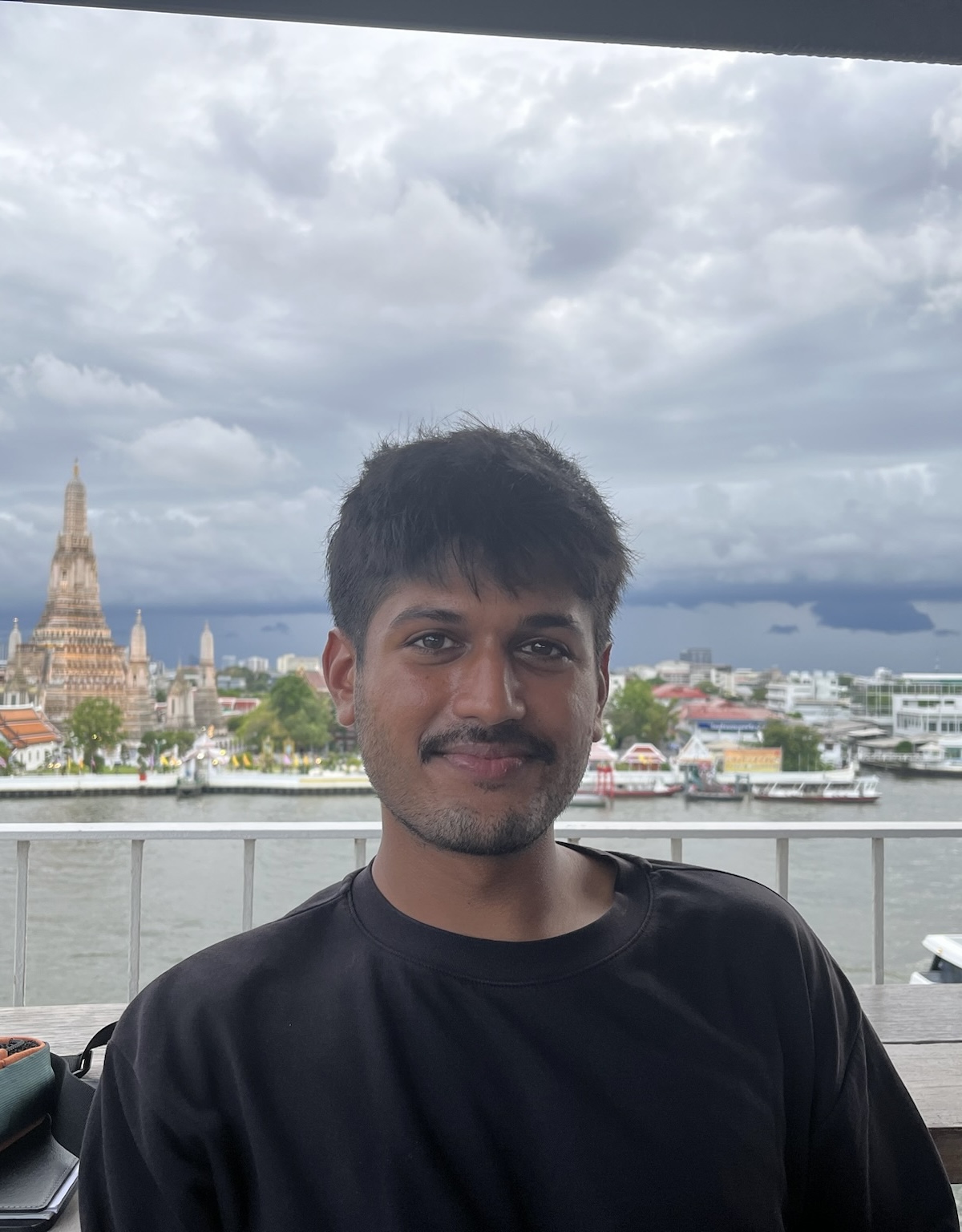
I’m interested in how task and data structure can promote the learning of representations that allow reuse/composition/transfer in different neural network architectures.
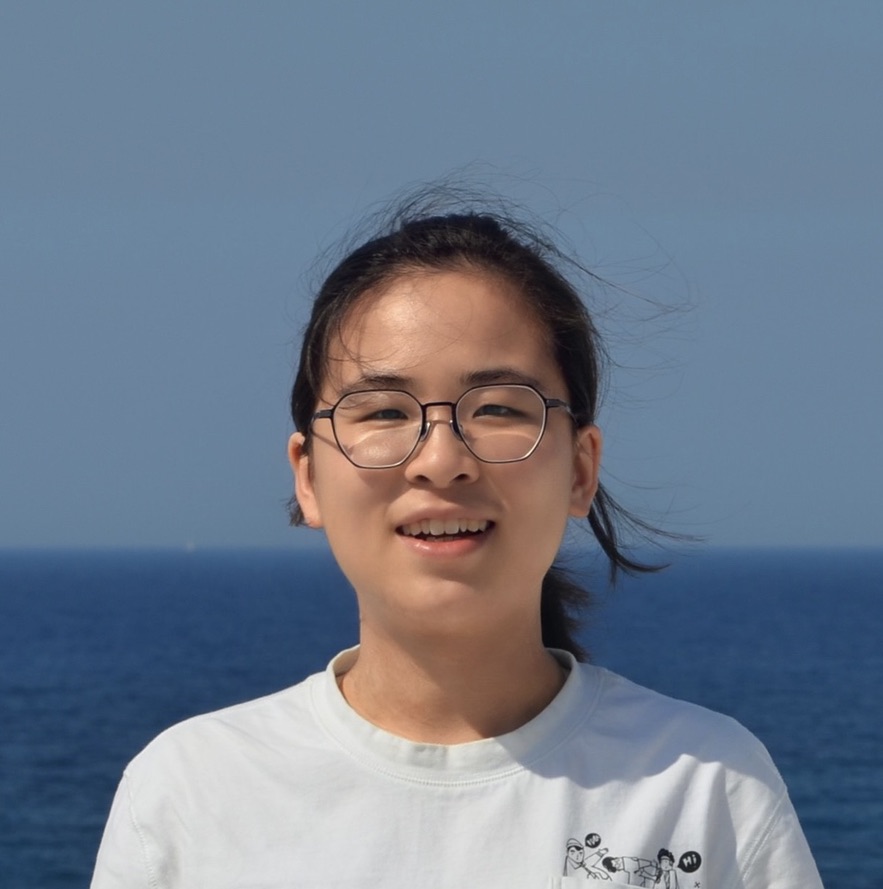
I am a PhD student at Gatsby co-advised by Peter Latham and Andrew Saxe.
I am interested in how neural networks with different architectures learn, including fully-connected, multimodal, and attention-based networks. Through theoretical approaches, I study how network architectures and data statistics shape the loss landscape and influence learning dynamics.
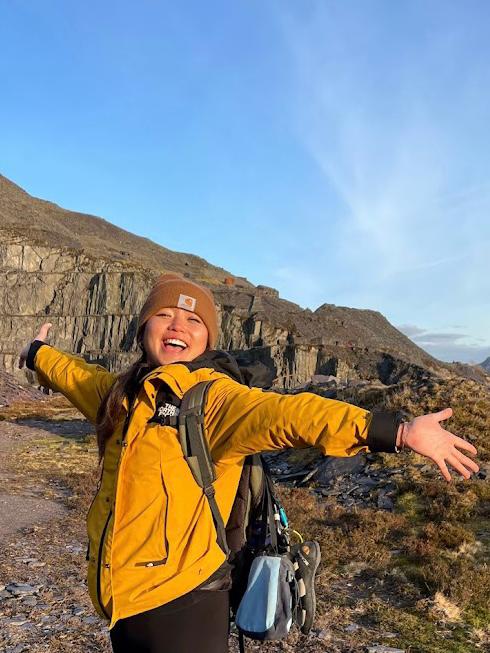
Compositional learning and inference is a core capability of intelligent agents. It allows agents to learn and perform complex tasks in a flexible way by combining lower level task and knowledge they already acquired.
My aim is to formulate a compositional cognitive process in biological agent, emulate it in artificial neural networks to establish an analytical understanding of the learning process.
To do this, I aim to study both computational/theoretical approach to describe a compositinal learning and experimental approach to figure out how biological agents represent and solve compositionality underlying tasks.
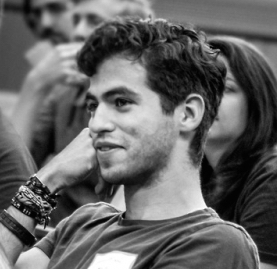
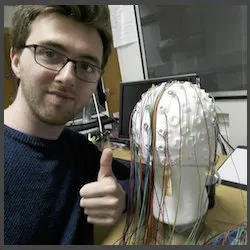
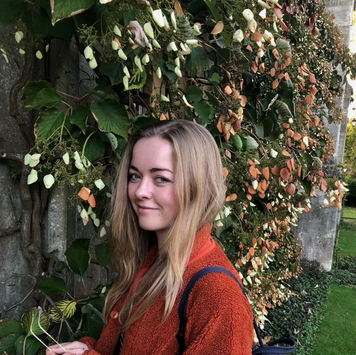
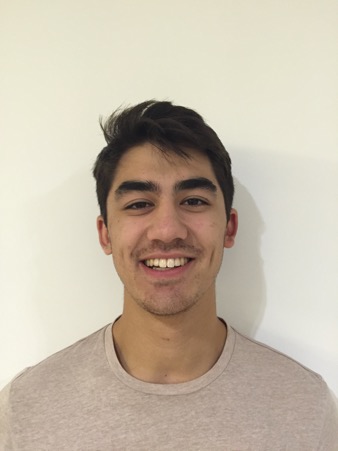
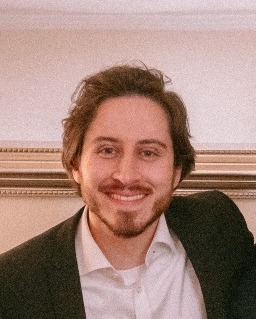
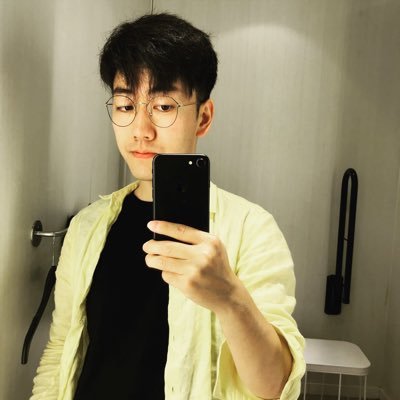
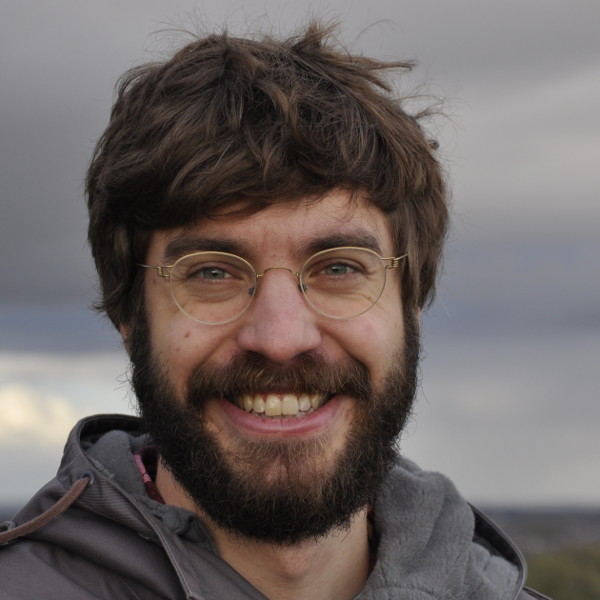
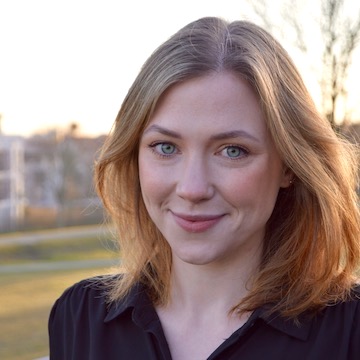
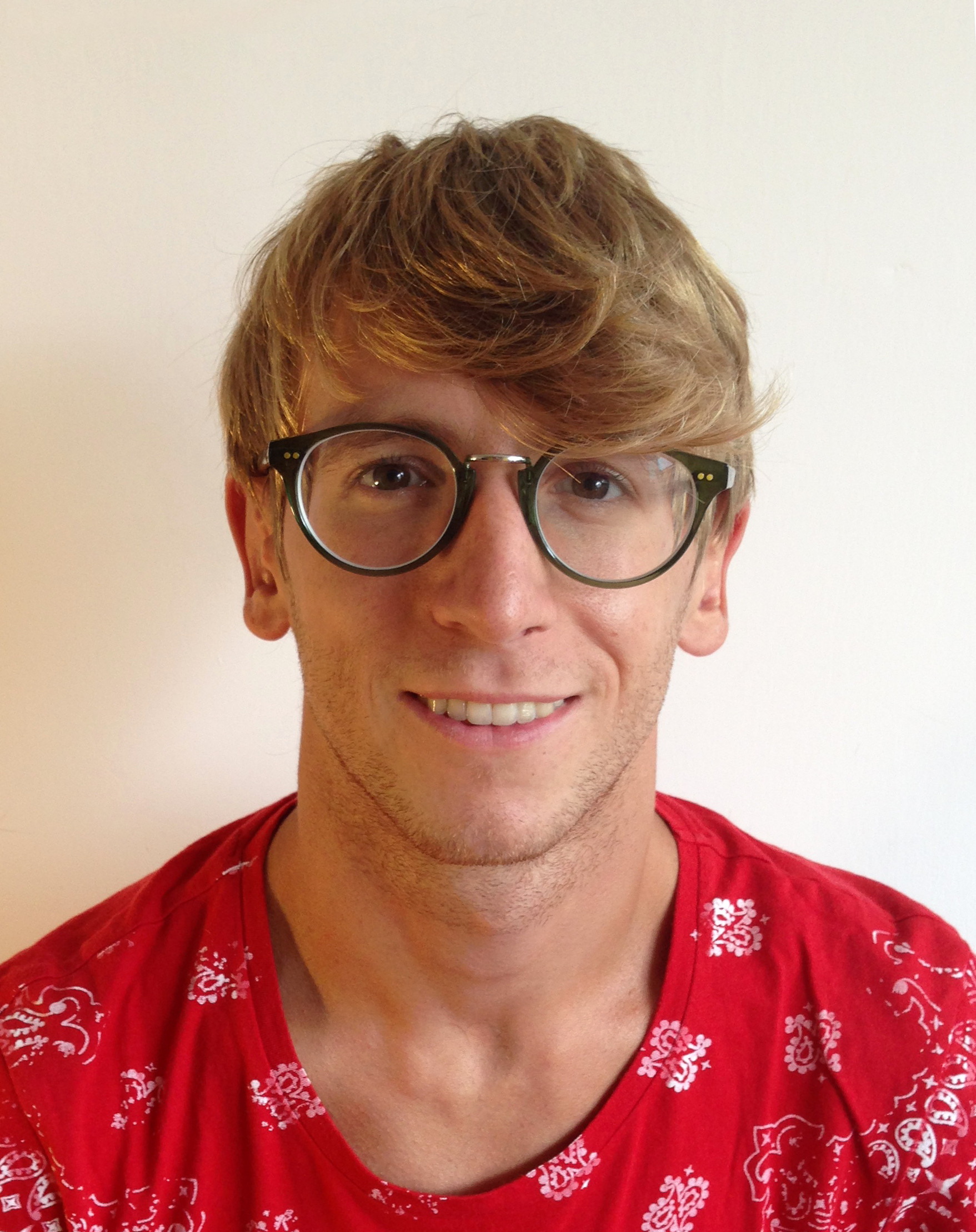
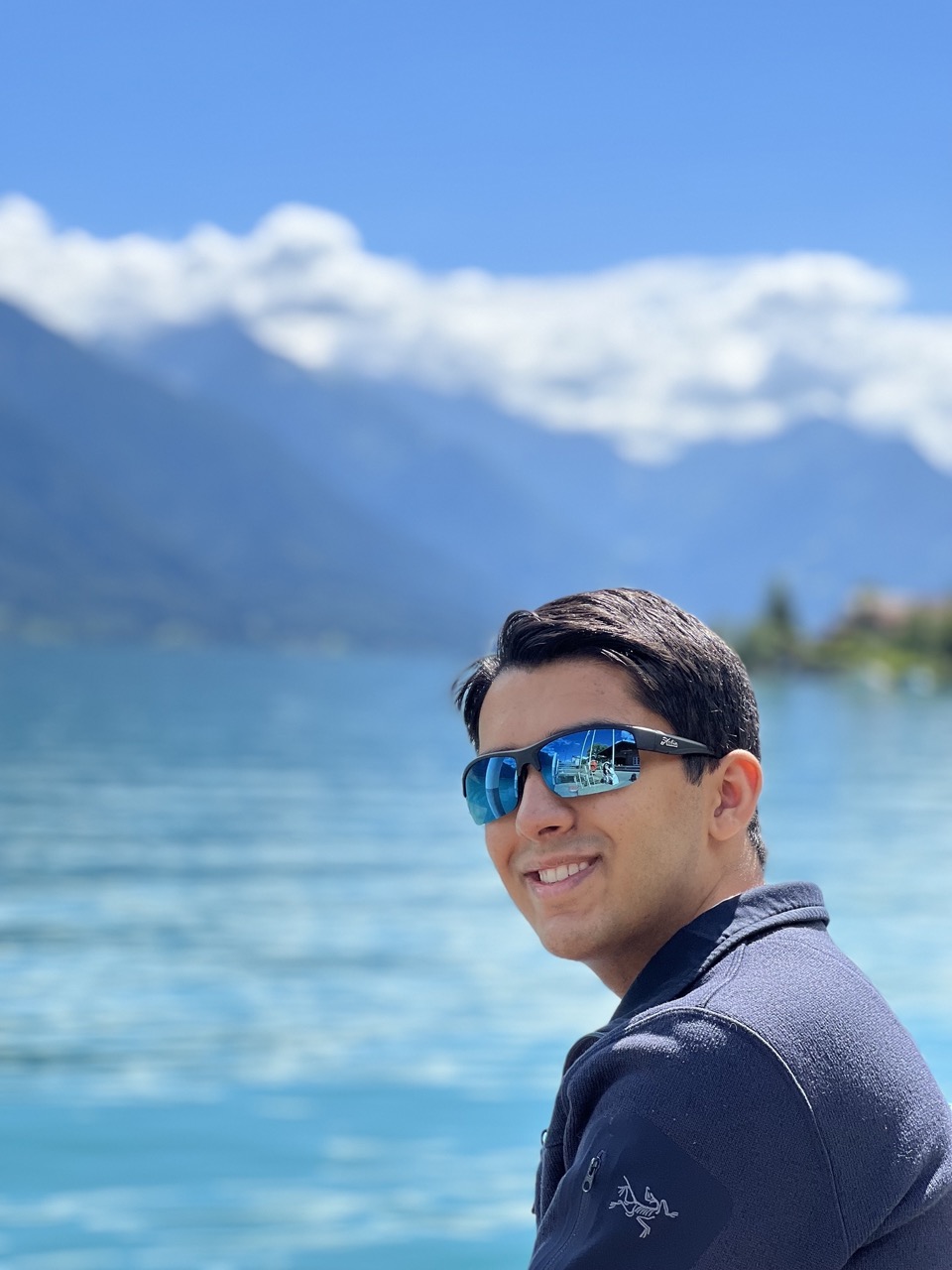